The implications of DeepSeek are worse than you think
And it should scare you.
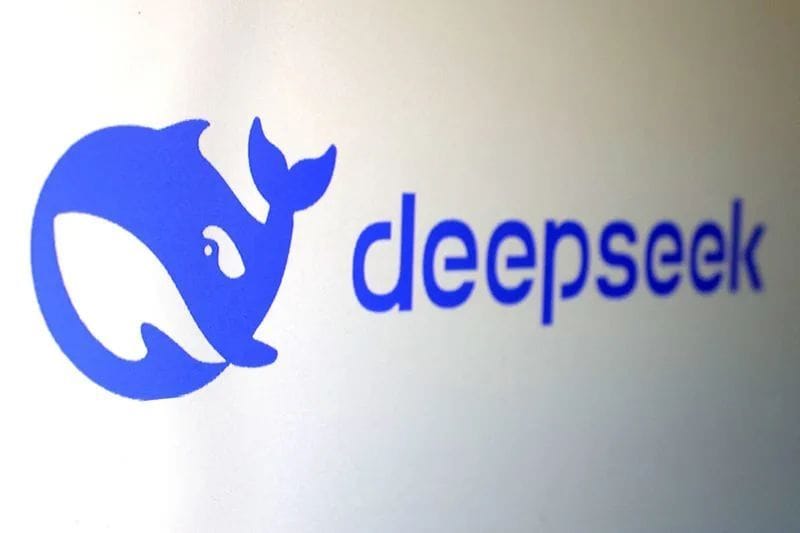
DeepSeek triggered a stock rout and fanned debate about US-China tech rivalry. If you look closer, I think the implications should scare you instead.
Fraction of the cost
China's DeepSeek released its new AI model last week. But it was its white paper about its R1 (reasoning) model that got top AI experts scrambling.
- Driven purely by Reinforcement Learning (RL).
- Meta's upcoming model might not be better than R1.
The older DeepSeek V3 (non-reasoning):
- Training cost of US$5.5M, a tenth of others.
- Beats OpenAI's GPT-4o in multiple benchmarks.
In a nutshell, DeepSeek managed to match up with or surpass the best Western models - and did it far more efficiently.
And DeepSeek released everything under the generous MIT license, including training secrets - far more than Meta's (not really open) open-source Llama.
And if DeepSeek shared so much voluntarily, what other tricks does the team have up its collective sleeve?
Market reactions
The market reacted with investors dumping tech stocks, wiping some US$593 billion off Nvidia - a record one-day loss for a Wall Street stock.
Why the selloff?
- The premise of AI had long focused on building ever-larger AI infrastructure to achieve better outcomes.
- However, DeepSeek's development of V3 and R1 with far fewer resources puts that narrative in doubt.
Despite neutral or even sanguine statements by tech leaders so far, there is no question at all that DeepSeek's achievements have unnerved them.
For instance, Meta has reportedly assembled four "war rooms" of engineers to respond to DeepSeek's breakthrough, according to The Information.
Continuous cycles of innovation
The release of DeepSeek R1 got me thinking.
The first x86 microprocessor was released in 1978. Today, chip makers continue to release new product families annually.
And microchips continue to get better. With the latest Nvidia GPU containing some 208 billion transistors, it won't be long before we cross the trillion-transistor mark.
Last year, when I floated the possibility of AI development plateauing, I said that the true impact of AI will take many years to catch up.
But what if AI never stops getting better? Year after year; decade after decade, we develop:
- Better chips.
- New algorithms.
- Larger AI models.
- Improved AI techniques.
Sure, AI output is often considered mediocre compared to top writers or coders today. But surely this won't be true for much longer - not at the current rate of progress.
Where would that leave us?